Keynotes
Title: Personalized Hearing Loss Compensation for the Next-generation Hearables and Hearing Aids
Abstract: With recent advances in precision hearing diagnostics and machine-learning methods for audio signal processing, fully personalized audio processing for early-onset and standard hearing impairments become within reach. However, this innovation comes with several challenges: embedding biophysical (hearing-impaired) models within closed-loop systems for algorithm design remains computationally challenging, and neural-network-based audio processing comes with entirely different sound-quality challenges than encountered in standard hearing aids. In this keynote, I will introduce how novel diagnostic methods can be used to individualize the parameters of biophysical and NN-based models of human auditory signal processing, and how these models (CoNNear) can be embedded within differentiable closed-loop systems to develop end-to-end signal processing for hearing aids and hearables. I will show examples of the restoration quality of the resulting systems and how they can be further optimized for real-time processing and excellent sound quality. These translational steps will facilitate their integration with the next-generation embedded systems for hearables and hearing-aids.
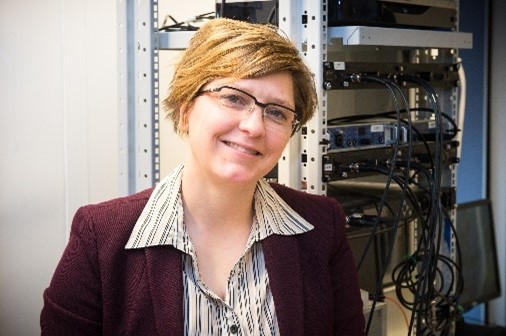
Biography: Prof. Dr. Sarah Verhulst Is Full Professor at Ghent University and leads the Hearing Technology lab. Her interdisciplinary research group works on the interface between auditory neuroscience, computational modeling and signal processing to innovate within the hearing-diagnostics and machine-hearing domains. She received two ERC grants and a recent EIC transition grant to leverage her scientific discoveries to the innovative technologies and market. She is a member of the Belgian Young Academy of Sciences, and a Fellow of the Acoustical Society of America.
Title: Next-Generation Speech Enhancement: Generative Diffusion Models and End-to-End Multichannel Filtering**
Abstract: In today’s digital age, devices such as telephones, video conferencing systems, and assistive listening devices are integral to daily communication, necessitating advanced speech enhancement and restoration algorithms to counter acoustic and transmission artifacts. Recent advances in machine learning have led to significant enhancements in both single-channel and multichannel speech enhancement techniques. In this presentation, we share our latest research on generative diffusion models for single-channel speech enhancement that enhance the naturalness of sound as perceived by listeners and provide superior generalization capabilities compared to traditional predictive methods. These models are effectively utilized across various restoration tasks including noise reduction, dereverberation, and bandwidth extension. We will also address ongoing challenges such as hallucinations at negative SNRs and the high computational demands of these models. Furthermore, we will present our investigation into how relatively small deep neural networks can substantially enhance speech quality when multiple microphones are available. Moving beyond conventional methods of separate spatial and spectral filtering, we have developed a joint nonlinear spatial-spectral filter trained in an end-to-end manner. Our results demonstrate that employing multiple microphones along with compact neural networks for this joint spatial-spectral filtering results in exceptional quality. This approach particularly excels in complex scenarios where the number of sound sources is larger than the number of microphones, considerably outperforming conventional methods. At the same time, the joint filter consists of a small neural architecture that can be implemented causally and is thus readily capable of real-time operation.
Biography: Timo Gerkmann (timo.gerkmann@uni-hamburg.de) is a Professor for Signal Processing at the Universität Hamburg, Germany. He has held positions with Technicolor Research & Innovation, University of Oldenburg, Germany, KTH Royal Institute of Technology, Stockholm, Sweden, Ruhr-Universität Bochum, Germany, and Siemens Corporate Research, Princeton, NJ, USA. His research interests include statistical signal processing and machine learning for speech and audio applied to communication devices, hearing instruments, audio-visual media, and human-machine interfaces. He was the recipient of the VDE ITG award 2022. He served in the IEEE Signal Processing Society Technical Committee on Audio and Acoustic Signal Processing and is currently a Senior Area Editor of the IEEE/ACM Transactions on Audio, Speech and Language Processing.
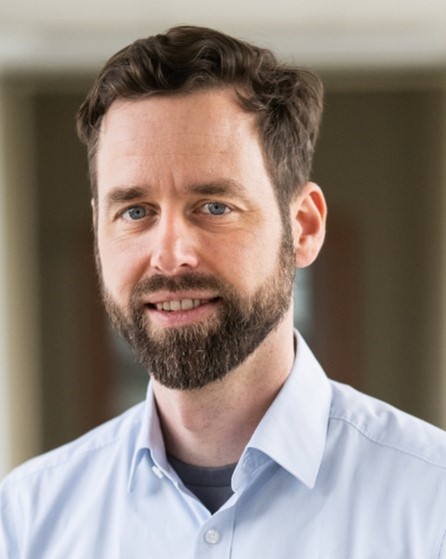
Title: New tools for Spatial Acoustic Signal Processing for Applications with Low SNR
Abstract: In this talk, I will discuss two recently introduced signal processing concepts and their example applications in problems involving propagation of signals over space with low SNR conditions. The first concept is the Relative Transfer Matrix (ReTM) which is a generalization of the Relative Transfer Function (ReTF) for multiple simultaneously active sound sources. We allocate receivers into two multichannel groups and formulate the Relative Transfer Matrix to describe the spatial acoustic channel between them. The second concept is called the Point Neuron Learning, a new physics-informed neural network (PINN) architecture that embeds the fundamental solution of the wave equation into the network architecture to strictly satisfy the wave equation. The point neuron learning method can model an arbitrary sound field based on microphone observations without any dataset, directly processes complex numbers and offers better interpretability and generalizability. I will illustrate the applications of ReTM and Point Neuron Learning in drone audition and speech enhancement in noisy reverberant rooms.
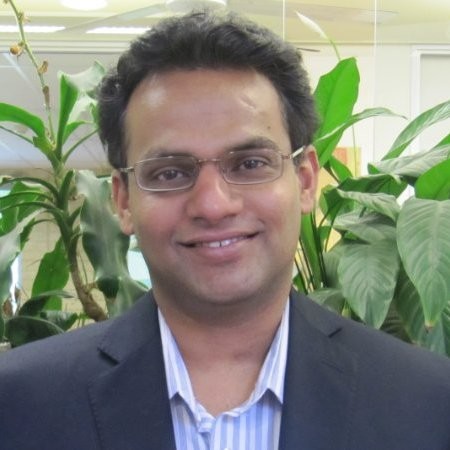
Biography: Thushara Abhayapala is a full Professor at the Australian National University (ANU), Canberra, Australia. He received the B.E. degree in engineering and the Ph.D. from ANU, in 1994 and 1999, respectively. He held several leadership positions, including the Deputy Dean with the ANU College of Engineering and Computer Science from 2015 to 2019, and Head of the ANU Research School of Engineering from 2010 to 2014. His research interests include the areas of spatial audio and acoustic signal processing, and multichannel signal processing. Among many contributions, he is one of the first researchers to use spherical harmonic based Eigen-decomposition in microphone arrays and to propose the concept of spherical microphone arrays and was one of the first to show the fundamental limits of spatial sound field reproduction using arrays of loudspeakers and spherical harmonics. He was the Co-chair of IEEE WASPAAA 2021. He was an Associate Editor for IEEE/ACM Transactions on Audio, Speech, and Language Processing. From 2011 to 2016, he was a Member of the Audio and Acoustic Signal Processing Technical Committee of the IEEE Signal Processing Society. He is a Fellow of IEEE and a Fellow of Engineers Australia.